Recommender Systems Should Drive Feeds That Consider Long-term Value to People
- 24 hours ago
- 4 min read
Issue #104
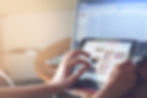
by Gary Marchionini (UNC School of Information & Library Science)
A March 2025 report from the Knight Georgetown Institute entitled “Better Feeds: Algorithms that put People First—A How-To Guide for Platforms and Policymakers”¹ integrates inputs from an impressive team of experts on recommendation systems that power social media, search, entertainment, and most e-commerce services. The report is organized into 6 sections and includes a useful abstract and background to frame the problem. One section provides a primer on recommendation systems for lay audiences, and the second section explains research on how and why today’s recommendation algorithms are tuned to maximize predicted user engagement as the key signal for what users experience in their information feeds rather than signals that benefit people over time. Another section overviews government and corporate policy in the US and EU, and the last three sections present guidelines for national and global policy makers and for designers and product teams. Although the policy discussions and guidelines have US and EU examples, I believe that information professionals around the world will benefit from the ideas in this report. Equally importantly, I believe we have crucial roles to play in making these systems more helpful to humanity.
Recommendation algorithms have been designed to maximize advertising and sales revenue streams, and they have been wildly successful in generating enormous advertising and direct sales revenue for platforms. They do so by using personalized feeds for users that reinforce impulse and emotional response. These feeds ultimately lead to a wide variety of individual and societal side effects, including personal angst; social disruptions; and local and global debates, legislation, and reactions. The authors argue that it does not have to be this way---that thoughtful design and policies can lead to algorithms that are satisfying and helpful to people over long periods of time, while also benefitting the companies and institutions that use them over the long term.
The report summarizes the thorny and evolving policy environment in the US and EU. The EU’s Digital Services Act foregrounds transparency, choice, and risk assessment at a general level. For example, the DSA requires platforms to offer users an alternative algorithm to the predicted engagement model.
This is an important step toward transparency; however, today’s implementations typically offer only a chronological feed that users often tire of and revert to the default predicted engagement feed. The DSA is a good start, and we must continue to develop other alternative feed models that are user-centric and make the settings and controls easy to understand and use. US legislative policies are mired in debates between innovation, immunity from liability, and the First Amendment rights to free speech, resulting in a plethora of legislation and court challenges with child safety prominent in many of the legislative discussions and proposals.
The report provides an interesting set of recommendations for system design, implementation, and policy. For algorithm design they look beyond chronological feeds as the solution. Chronological feeds are suboptimal for users because they may amplify non-relevant content, incentivize spam-like postings, are not workable for all kinds of platforms, and decrease positive engagement. The authors recommend three recommendations tuned to signals that are not limited to engagement metrics. These include a) Bridging across communities and points of view (diversity of engagement and commentary is a positive signal rather than ‘more like I like’). b) Surveys about what people explicitly like and do not like about items and the overall experience—clearly, these take time for users to complete and depend on good design as well as user education to understand the benefits of investing that time. c) Incorporating content quality factors (e.g., toxic language, source reputation, etc.) without descending into the morass of content moderation debates on subjective factors. The report recommends that platforms make these alternative long-term user value-oriented feeds the default setting rather than existing personalized predicted user engagement feeds.
These design issues are squarely within the scope of information science research and practice. Information scholars have important ideas and practices to contribute to recommendation system design that is human-centric and long-term value driven rather than short-term platform driven algorithms and metrics. For example, although it is more expensive to gather, direct expressions of user preferences are far superior to inferred preference data based on actions (e.g., click, like) executed by a group of users with some assumed similarity to an individual (e.g., location, age). Information scholars are skilled at developing elicitations (e.g., surveys, easy to understand and use control settings) that are effective and inviting and we can educate and encourage people to understand that taking a bit of time to provide explicit feedback or to be thoughtful about what default and optional controls are selected is in their best long-term interest. These skills will be valuable if platforms begin to follow the advice of reports like this to benefit long-term user value that also in turn benefits the platform’s long-term success.
Another design recommendation is to encourage more design transparency, including public disclosure of what input data is used and what weights are assigned (acknowledging that some of these techniques are competitive advantage---weights for example could be reported as quartiles rather than specific settings). Likewise, platforms should disclose metrics used for long-term user value as well as metrics used to evaluate internal product teams. Information scholars’ expertise in open systems can be helpful in organizing and reporting disclosures, and especially in assuming roles as data auditors for these metrics and disclosures.
Other recommendations in the report are to use long-term holdout experiments to assess user preferences and performance rather than only simple, short-term A-B testing that is the standard today. Platforms should regularly report retention metrics, user satisfaction, and summaries of harms or benefits. Additionally, the report recommends that platforms regularly publish samples of highly disseminated content and samples of highly consumed content and publicly disclose aggregate harms to high-risk populations.
This report is one of the many timely and thoughtful considerations of the current state of the digital information exposome. We increasingly live, work, and play in both analog and digital worlds and information scholars are well-prepared to participate in investigating effects and impacts from a human-centric perspective and to help humanity understand and thrive in these environments.
1: Moehring, A., Cooper, A., Narayanan, A., Ovadya, A., Redmiles, E., Allen, J., . . . Arnao, Z. (2025). Better Feeds: Algorithms That Put People First. Knight–Georgetown Institute. https://kgi.georgetown.edu/research-and-commentary/better-feeds/
Feature Stories solely reflect the opinion of the author.