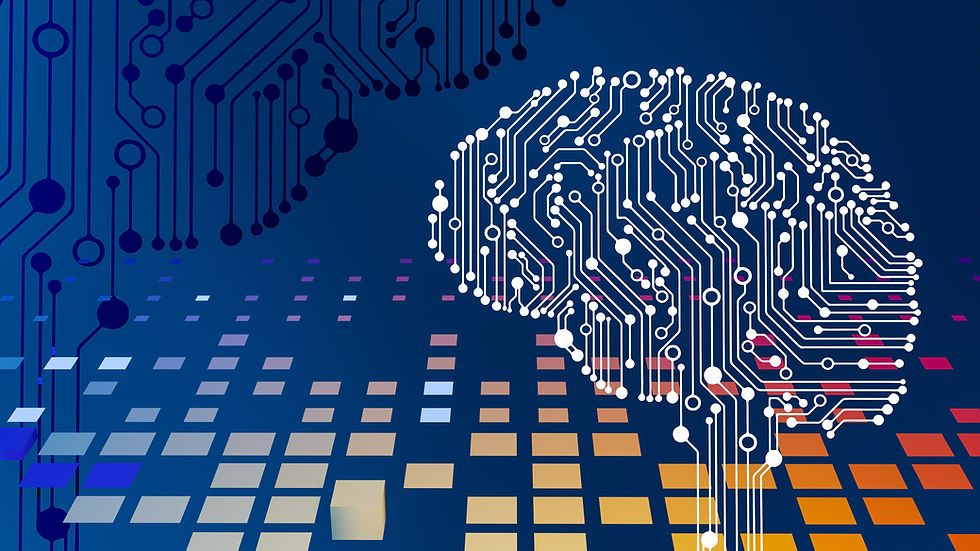
Carnegie Mellon University, Heinz College of Information Systems and Public Policy
Behind all the histrionics of generative artificial intelligence—behind all the poetry and coding, the jailbreaks and hallucinations—lies a math problem.
Generative AI, like OpenAI’s ChatGPT and Google’s Bard, relies on statistical probability. Today’s computers are powerful enough to train large language models on billions of pages of text. The models can detect the patterns of language well enough to respond with startling lucidity, but they do not truly understand the subject matter. Given a word or letter, the models reference their training data and determine the word or set of words most likely to come next.
It’s a math problem. But left unchecked, it could be a real problem.
“I don't think we all need to run around in complete panic, but it's very good if some people think about this more,” said Vincent Conitzer, a member of the Block Center for Technology and Society’s Advisory Council and the director of CMU’s Foundations of Cooperative AI (FOCAL) Lab. “And I think it's more of a broader problem that we don't really understand.”
Because we cannot predict the extent of AI’s proliferation, we cannot guess the full extent of its potential pitfalls. At this stage in its development, three categories of risk have made themselves apparent.
The system is working … right?
The first category involves circumstances in which the AI functions properly, but still creates negative outcomes. “Functions properly” is a loaded phrase, because the algorithm can synthesize a query, produce a relevant response and still cause harm. The creators of large language models attempt to mitigate harmful responses with a method called Reinforcement Learning from Human Feedback.
“There are two components to these systems,” said Rayid Ghani, a Distinguished Career Professor at the Heinz College of Information Systems and Public Policy and in CMU’s Machine Learning Department. “Component one is, it takes all the data that's on the Internet and predicts the next word. And we kind of understand what that is. Component two is, it's been trained from human feedback for the last few years, and nobody outside OpenAI knows how that is done and what its impact is.”
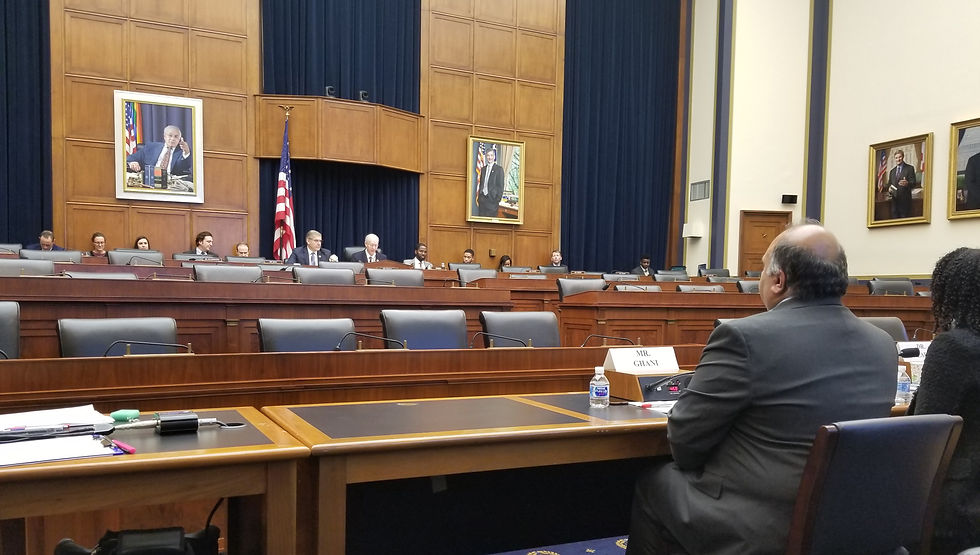
Fair and Equitable - Professor Rayid Ghani uses machine learning, AI and data science to solve high-impact social good and public policy problems in a fair and equitable way.
Ghani, the former Chief Scientist of President Barack Obama’s 2012 re-election campaign, studies these issues closely. He teaches a Machine Learning for Public Policy Lab, helping Heinz College’s Master of Science in Public Policy and Management: Data Analytics and Master of Information Systems Management students marry the technical know-how with the policy needed to govern it. He also started the Data Science for Social Good Fellowship, which trains computer scientists, statisticians and social scientists from around the world to work on data science problems with social impact.
Because humans wrote the words used to train these large language models, and because humans, unfortunately, are biased in many harmful ways, the chatbot could produce responses that also contain those biases. And even if people use large language models to help them in ethical, legal ways, how much of future models’ training data will consist of text a former model wrote?
AI can help people at work by reducing errors, automating monotonous tasks, and allowing humans to focus on the more creative or interpersonal aspects of their jobs. But in the short term, it will probably put some people out of work, or squeeze portions of the job market. Improved search, summarization and writing ability could reduce billable hours for lawyers, for example, or decrease the need for paralegals. Ghani compared this result to what happened to typists and secretaries as technology improved.
“When technology came, it created more jobs, but not for them,” he said. “Because the people displaced were not the people who were upskilled. It created a job for somebody else. And that’s what's going to happen here. The person who's displaced isn't going to become an AI programmer.”
It’s a People Problem
No matter how noble the mission of generative AI, human nature ensures that people will misuse it, and here we find the second area of concern: situations when the model behaves properly, but those who use it do not.
ChatGPT has already been conned into creating polymorphic malware, which shape-shifts to avoid detection inside a target network. Humans produce and distribute plenty of misinformation already in an effort to sway public opinion, but generative AI can do it faster and at scale. What it can also do, which is even more dangerous, is create images, videos and audio, known as deepfakes, that make it look and sound like someone is saying or doing something they aren’t. So far it’s been the Pope in a big jacket and Jerry Seinfeld in Pulp Fiction. What happens when it’s Joe Biden declaring war on Russia or someone making a withdrawal from your bank account with your voice?

AI as a FOCAL Point - Vincent Conitzer directs Carnegie Mellon's Foundations of Cooperative AI (FOCAL) Lab.
“It ain’t what you don’t know that gets you into trouble. It’s what you know for sure that just ain’t so.” ~ Mark Twain
Then we come to the third category, which will exacerbate everything in the first two. These are issues created when the AI makes mistakes.
Sometimes, large language models will “hallucinate,” the fancy term for making stuff up. It’ll sound credible, written with perfect grammar, and may contain kernels of truth, but it’ll be pure fiction. And just like the issues with bias, it'll have a sheen of accuracy and impartiality; it came from a computer, after all.
“Everything it generates is plausible,” Ghani said. “And that's exactly what it's designed to do, is generate plausible things, rather than factually correct things, because it doesn't know the difference between factually correct and plausible.”
The threats posed by generative AI have the attention of the White House, which in October 2022 published a blueprint for an AI Bill of Rights. The proposal identifies data privacy, protection from discrimination, safe and effective systems, proper explanation, and human alternatives as the pillars of responsibly deployed AI. The Department of Commerce convened a National Artificial Intelligence Advisory Committee, on which Heinz College Dean Ramayya Krishnan sits. And in late May, more than 350 A.I. executives and researchers, including Conitzer and the CEOs of OpenAI and Google's DeepMind, signed their name to a statement that read, “Mitigating the risk of extinction from AI should be a global priority alongside other societal-scale risks such as pandemics and nuclear war.”
Smart people are asking the right questions. The question now is whether they can find a consensus quickly enough to keep pace with the ever-evolving technology, and more important still, if that consensus can become binding and enforceable.
If only that were as simple as a math problem.
Comments